強(qiáng)化學(xué)習(xí)與最優(yōu)控制
鏈接:https://pan.baidu.com/s/1wgyQz-jpgF32a-lkQzuV7A?pwd=8xc7?
提取碼:8xc7
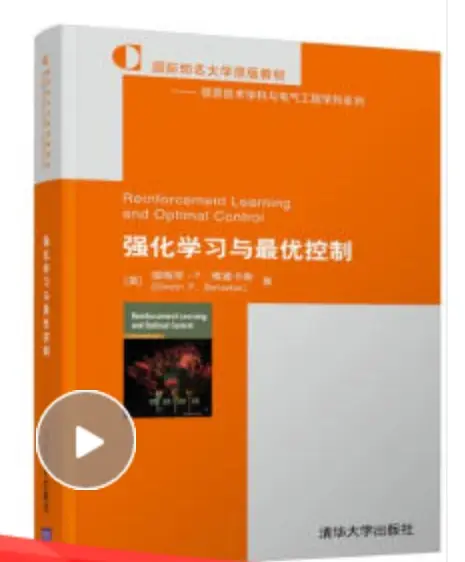
Dimitri P. Bertseka,美國MIT終身教授,美國國家工程院院士,清華大學(xué)復(fù)雜與網(wǎng)絡(luò)化系統(tǒng)研究中心客座教授,電氣工程與計(jì)算機(jī)科學(xué)領(lǐng)域國際知名作者,著有《非線性規(guī)劃》《網(wǎng)絡(luò)優(yōu)化》《凸優(yōu)化》等十幾本暢銷教材和專著。本書的目的是考慮大型且具有挑戰(zhàn)性的多階段決策問題,這些問題原則上可以通過動(dòng)態(tài)規(guī)劃和最優(yōu)控制來解決,但它們的精確解決方案在計(jì)算上是難以處理的。本書討論依賴于近似的解決方法,以產(chǎn)生具有足夠性能的次優(yōu)策略。這些方法統(tǒng)稱為增強(qiáng)學(xué)習(xí),也可以叫做近似動(dòng)態(tài)規(guī)劃和神經(jīng)動(dòng)態(tài)規(guī)劃等。
本書的主題產(chǎn)生于最優(yōu)控制和人工智能思想的相互作用。本書的目的之一是探索這兩個(gè)領(lǐng)域之間的共同邊界,并架設(shè)一座具有任一領(lǐng)域背景的專業(yè)人士都可以訪問的橋梁。
內(nèi)容簡介
《強(qiáng)化學(xué)習(xí)與最優(yōu)控制(英文版)》的目的是考慮大型且具有挑戰(zhàn)性的多階段決策問題,這些問題原則上可以通過動(dòng)態(tài)規(guī)劃和優(yōu)控制來解決,但它們的解決方案在計(jì)算上是難以處理的?!稄?qiáng)化學(xué)習(xí)與最優(yōu)控制(英文版)》討論依賴于近似的解決方法,以產(chǎn)生具有足夠性能的次優(yōu)策略。這些方法統(tǒng)稱為增強(qiáng)學(xué)習(xí),也可以叫做近似動(dòng)態(tài)規(guī)劃和神經(jīng)動(dòng)態(tài)規(guī)劃等。《強(qiáng)化學(xué)習(xí)與最優(yōu)控制(英文版)》的主題產(chǎn)生于優(yōu)控制和人工智能思想的相互作用。《強(qiáng)化學(xué)習(xí)與最優(yōu)控制(英文版)》的目的之一是探索這兩個(gè)領(lǐng)域之間的共同邊界,并架設(shè)一座具有任一領(lǐng)域背景的人士都可以訪問的橋梁。
作者簡介
Dimitri P. Bertseka,美國MIT終身教授,美國國家工程院院士,清華大學(xué)復(fù)雜與網(wǎng)絡(luò)化系統(tǒng)研究中心客座教授。電氣工程與計(jì)算機(jī)科學(xué)領(lǐng)域國際知名作者,著有《非線性規(guī)劃》《網(wǎng)絡(luò)優(yōu)化》《凸優(yōu)化》等十幾本暢銷教材和專著。
目錄
Contents
1. Exact Dynamic Programming
1.1. DeterministicDynamicProgramming . . . . . . . . . . . p. 2
1.1.1. DeterministicProblems . . . . . . . . . . . . . . p. 2
1.1.2. TheDynamicProgrammingAlgorithm . . . . . . . . p. 7
1.1.3. Approximation inValue Space . . . . . . . . . . . p. 12
1.2. StochasticDynamicProgramming . . . . . . . . . . . . . p. 14
1.3. Examples,Variations, and Simplifications . . . . . . . . . p. 18
1.3.1. Deterministic ShortestPathProblems . . . . . . . . p. 19
1.3.2. DiscreteDeterministicOptimization . . . . . . . . . p. 21
1.3.3. Problemswith aTermination State . . . . . . . . . p. 25
1.3.4. Forecasts . . . . . . . . . . . . . . . . . . . . . p. 26
1.3.5. Problems with Uncontrollable State Components . . . p. 29
1.3.6. PartialState Information andBelief States . . . . . . p. 34
1.3.7. LinearQuadraticOptimalControl . . . . . . . . . . p. 38
1.3.8. SystemswithUnknownParameters -Adaptive . . . . . .
Control . . . . . . . . . . . . . . . . . . . . . p. 40
1.4. ReinforcementLearning andOptimalControl - Some . . . . . .
Terminology . . . . . . . . . . . . . . . . . . . . . . p. 43
1.5. Notes and Sources . . . . . . . . . . . . . . . . . . . p. 45
2. Approximation in Value Space
2.1. ApproximationApproaches inReinforcementLearning . . . . p. 50
2.1.1. General Issues ofApproximation inValue Space . . . . p. 54
2.1.2. Off-Line andOn-LineMethods . . . . . . . . . . . p. 56
2.1.3. Model-Based Simplification of the Lookahead . . . . . .
Minimization . . . . . . . . . . . . . . . . . . . p. 57
2.1.4. Model-Free off-Line Q-Factor Approximation . . . . p. 58
2.1.5. Approximation inPolicy Space onTop of . . . . . . . .
ApproximationinValue Space . . . . . . . . . . . p. 61
2.1.6. When is Approximation in Value Space Effective? . . . p. 62
2.2. Multistep Lookahead . . . . . . . . . . . . . . . . . . p. 64
??ii
viii Contents
2.2.1. Multistep Lookahead and Rolling Horizon . . . . . . p. 65
2.2.2. Multistep Lookahead and Deterministic Problems . . . p. 67
2.3. Problem Approximation . . . . . . . . . . . . . . . . . p. 69
2.3.1. Enforced Decomposition . . . . . . . . . . . . . . p. 69
2.3.2. Probabilistic Approximation - Certainty Equivalent . . . .
Control . . . . . . . . . . . . . . . . . . . . . p. 76
2.4. Rollout and the Policy Improvement Principle . . . . . . . p. 83
2.4.1. On-Line Rollout for Deterministic Discrete . . . . . . . .
Optimization . . . . . . . . . . . . . . . . . . . p. 84
2.4.2. Stochastic Rollout and Monte Carlo Tree Search . . . p. 95
2.4.3. Rollout with an Expert . . . . . . . . . . . . . p. 104
2.5. On-Line Rollout for Deterministic Infinite-Spaces Problems - . . .
Optimization Heuristics . . . . . . . . . . . . . . . . p. 106
2.5.1. Model Predictive Control . . . . . . . . . . . . . p. 108
2.5.2. Target Tubes and the Constrained Controllability . . . . .
Condition . . . . . . . . . . . . . . . . . . . p. 115
2.5.3. Variants of Model Predictive Control . . . . . . . p. 118
2.6. Notes and Sources . . . . . . . . . . . . . . . . . . p. 120
3. Parametric Approximation
3.1. Approximation Architectures . . . . . . . . . . . . . . p. 126
3.1.1. Linear and Nonlinear Feature-Based Architectures . . p. 126
3.1.2. Training of Linear and Nonlinear Architectures . . . p. 134
3.1.3. Incremental Gradient and Newton Methods . . . . . p. 135
3.2. Neural Networks . . . . . . . . . . . . . . . . . . . p. 149
3.2.1. Training of Neural Networks . . . . . . . . . . . p. 153
3.2.2. Multilayer and Deep Neural Networks . . . . . . . p. 157
3.3. Sequential Dynamic Programming Approximation . . . . . p. 161
3.4. Q-Factor Parametric Approximation . . . . . . . . . . . p. 162
3.5. Parametric Approximation in Policy Space by . . . . . . . . .
Classification . . . . . . . . . . . . . . . . . . . . . p. 165
3.6. Notes and Sources . . . . . . . . . . . . . . . . . . p. 171
4. Infinite Horizon Dynamic Programming
4.1. An Overview of Infinite Horizon Problems . . . . . . . . p. 174
4.2. Stochastic Shortest Path Problems . . . . . . . . . . . p. 177
4.3. Discounted Problems . . . . . . . . . . . . . . . . . p. 187
4.4. Semi-Markov Discounted Problems . . . . . . . . . . . p. 192
4.5. Asynchronous Distributed Value Iteration . . . . . . . . p. 197
4.6. Policy Iteration . . . . . . . . . . . . . . . . . . . p. 200
4.6.1. Exact Policy Iteration . . . . . . . . . . . . . . p. 200
4.6.2. Optimistic and Multistep Lookahead Policy . . . . . . .
Iteration . . . . . . . . . . . . . . . . . . . . p. 205
4.6.3. Policy Iteration for Q-factors . . . . . . . . . . . p. 208
Contents i??
4.7. Notes and Sources . . . . . . . . . . . . . . . . . . p. 209
4.8. Appendix: MathematicalAnalysis . . . . . . . . . . . p. 211
4.8.1. Proofs for Stochastic ShortestPathProblems . . . . p. 212
4.8.2. Proofs forDiscountedProblems . . . . . . . . . . p. 217
4.8.3. ConvergenceofExact andOptimistic . . . . . . . . . .
Policy Iteration . . . . . . . . . . . . . . . . p. 218
5. Infinite Horizon Reinforcement Learning
5.1. Approximation in Value Space - Performance Bounds . . . p. 222
5.1.1. LimitedLookahead . . . . . . . . . . . . . . . p. 224
5.1.2. Rollout and Approximate Policy Improvement . . . p. 227
5.1.3. ApproximatePolicy Iteration . . . . . . . . . . . p. 232
5.2. FittedValue Iteration . . . . . . . . . . . . . . . . . p. 235
5.3. Simulation-BasedPolicy IterationwithParametric . . . . . . .
Approximation . . . . . . . . . . . . . . . . . . . . p. 239
5.3.1. Self-Learning andActor-CriticMethods . . . . . . p. 239
5.3.2. Model-Based Variant of a Critic-Only Method . . . p. 241
5.3.3. Model-FreeVariant of aCritic-OnlyMethod . . . . p. 243
5.3.4. Implementation Issues ofParametricPolicy . . . . . . .
Iteration . . . . . . . . . . . . . . . . . . . . p. 246
5.3.5. Convergence Issues ofParametricPolicy Iteration - . . . .
Oscillations . . . . . . . . . . . . . . . . . . . p. 249
5.4. Q-Learning . . . . . . . . . . . . . . . . . . . . . p. 253
5.4.1. Optimistic Policy Iteration with Parametric Q-Factor . . .
Approximation- SARSAandDQN . . . . . . . . p. 255
5.5. AdditionalMethods -TemporalDifferences . . . . . . . p. 256
5.6. Exact andApproximateLinearProgramming . . . . . . p. 267
5.7. Approximation inPolicy Space . . . . . . . . . . . . . p. 270
5.7.1. Training byCostOptimization -PolicyGradient, . . . . .
Cross-Entropy,andRandomSearchMethods . . . . p. 276
5.7.2. Expert-BasedSupervisedLearning . . . . . . . . p. 286
5.7.3. ApproximatePolicy Iteration,Rollout, and . . . . . . .
ApproximationinPolicySpace . . . . . . . . . . p. 288
5.8. Notes and Sources . . . . . . . . . . . . . . . . . . p. 293
5.9. Appendix: MathematicalAnalysis . . . . . . . . . . . p. 298
5.9.1. Performance Bounds for Multistep Lookahead . . . . p. 299
5.9.2. Performance Bounds for Rollout . . . . . . . . . . p. 301
5.9.3. Performance Bounds for Approximate Policy . . . . . . .
Iteration . . . . . . . . . . . . . . . . . . . . p. 304
6. Aggregation
6.1. AggregationwithRepresentativeStates . . . . . . . . . p. 308
6.1.1. Continuous State and Control Space Discretization . p. 314
6.1.2. Continuous State Space - POMDP Discretization . . p. 315
?? Contents
6.2. AggregationwithRepresentativeFeatures . . . . . . . . p. 317
6.2.1. Hard Aggregation and Error Bounds . . . . . . . . p. 320
6.2.2. AggregationUsingFeatures . . . . . . . . . . . . p. 322
6.3. Methods for Solving theAggregateProblem . . . . . . . p. 328
6.3.1. Simulation-BasedPolicy Iteration . . . . . . . . . p. 328
6.3.2. Simulation-Based Value Iteration . . . . . . . . . p. 331
6.4. Feature-BasedAggregationwith aNeuralNetwork . . . . p. 332
6.5. BiasedAggregation . . . . . . . . . . . . . . . . . . p. 334
6.6. Notes and Sources . . . . . . . . . . . . . . . . . . p. 337
6.7. Appendix: MathematicalAnalysis . . . . . . . . . . . p. 340
References . . . . . . . . . . . . . . . . . . . . . . . p. 345
Index . . . . . . . . . . . . . . . . . . . . . . . . . . p. 369
查看全部↓
前言/序言
Preface
Turning to the succor of modern computing machines, let us
renounce all analytic tools.
Richard Bellman [Bel57]
From a teleological point of view the particular numerical solution
of any particular set of equations is of far less importance than
the understanding of the nature of the solution.
Richard Bellman [Bel57]
In this book we consider large and challenging multistage decision problems,
which can be solved in principle by dynamic programming (DP for short),
but their exact solution is computationally intractable. We discuss solution
methods that rely on approximations to produce suboptimal policies with
adequate performance. These methods are collectively known by several
essentially equivalent names: reinforcement learning, approximate dynamic
programming, and neuro-dynamic programming. We will use primarily the
most popular name: reinforcement learning.
Our subject has benefited greatly from the interplay of ideas from
optimal control and from artificial intelligence. One of the aims of the
book is to explore the common boundary between these two fields and to
form a bridge that is accessible by workers with background in either field.
Another aim is to organize coherently the broad mosaic of methods that
have proved successful in practice while having a solid theoretical and/or
logical foundation. This may help researchers and practitioners to find
their way through the maze of competing ideas that constitute the current
state of the art.
There are two general approaches for DP-based suboptimal control.
The first is approximation in value space, where we approximate in some
way the optimal cost-to-go function with some other function. The major
alternative to approximation in value space is approximation in policy
查看全部↓